Imagine that you are considering buying stock in a company. You know what its current value is, and you suspect that you could make a healthy return on your investment. But this stock is very volatile: some days up, some days down. Yes, you could make a lot of money, but you could also lose a lot of money. There is a clear reward, but also a lot of risk.
Many decisions are like this. The can of tomato paste on clearance at the grocery store is a fantastic bargain if it has not gone bad, but if it has, you have thrown away your money.
Decisions like these are a classic situation considered by economists. New research from the lab of John O'Doherty, Caltech's Fletcher Jones Professor of Decision Neuroscience and an affiliate faculty member of the Tianqiao and Chrissy Chen Institute for Neuroscience, aims to understand how the brain implements these kinds of decisions by testing a computational model that proposes how representations of reward and risk are built from experience. The neural processing of reward and risk was previously studied at Caltech via a technique called functional magnetic resonance imaging (fMRI), which measures changes in blood flow inside the brain. Researchers found that a region of the brain called the anterior insula is activated when people assess risk and process uncertainty.
In a new study, electrodes implanted deep within the brains of patients (for unrelated therapeutic purposes) allowed O'Doherty and his team to obtain even more precise measurements of brain activity during decision-making. The work revealed that, as expected, the so-called reward prediction error (the difference between the expected value and the observed value) appears first and is followed by the risk prediction error (the difference between the expected uncertainty and the actual uncertainty), which relied on the same neural processes as the reward prediction error. Both signals were found in the anterior insula. These findings suggest that the reward prediction error is used to calculate the risk prediction error, which can then be used to learn to assess riskiness, which is a necessary guide to decision-making.
The work was published in the March 9, 2024, issue of Nature Communications.
Vincent Man, a senior postdoctoral scholar research associate in neuroscience and a co-author of the paper, explains: "fMRI is great at telling us where in the brain something is happening, but it is limited in terms of telling us when things happen, at least on the fast timescales at which we think these neural processes unfold."
For this study, patients being evaluated for epilepsy were recruited at the University of Iowa Hospitals and Clinics. To monitor their seizure activity, these individuals had electrodes implanted deep in key regions of their brain, including in the anterior insula, which allowed researchers to detect neural activity at a microsecond timescale that is not possible with fMRI.
Then, the participants played a very simple card game using 10 playing cards numbered from ace to 10, with the ace counting as one. They were asked to predict, sight unseen, if the second card would be higher or lower in value than the first card. Since neither card was visible, this was always a completely random guess. After the first card was shown, participants would get some information about how accurate their guess might be. For example, if they predicted that the second card would be lower, and the first card was a 10, they would know immediately that their guess was correct. If the first card was an ace, they would know they were wrong. But if the first card was a five, the outcome remained uncertain until the second card was revealed.
"Basically, with this game we are drawing an arc from no uncertainty to maximal uncertainty," explains Man, who works in O'Doherty's lab. "The computational model predicts that you make one computation and form an expectation about risk. When you see card two, there is a second computation to assess the expected risk." The computations used to make these predictions are identified as the reward prediction error (RePE)—the process of updating between an expected reward and an observed reward (the actual card drawn), and the risk prediction error (RiPE)—the process of assessing the expected risk with respect to the observed risk.
Activity detected in the anterior insula during these games showed exactly this two-step process following the display of card two: reward prediction evaluation first, followed by risk prediction error evaluation.
"We're validating a theoretical idea about the relationship between reward and risk and how they relate to each other," Man says. "The fact that the neural signature is consistent with the theory is nice; it grounds the theory."
O'Doherty adds: "Determining how the brain generates these kinds of computations can help us ultimately build more accurate models of how the brain learns and make decisions, which could be useful not only for understanding how the brain works in general, but also, potentially, for understanding how these processes might go wrong in diseases such as problem gambling, addiction, or other psychiatric disorders."
The paper is titled "Temporally Organized Representation of Reward and Risk in the Human Brain." The authors are Man, O'Doherty, and Jeffrey Cockburn of Caltech; Oliver Flouty of the University of South Florida; and Phillip E. Gander, Masahiro Sawada, Christopher K. Kovach, Hiroto Kawasaki, Hiroyuki Oya, and Matthew A. Howard III of the University of Iowa.
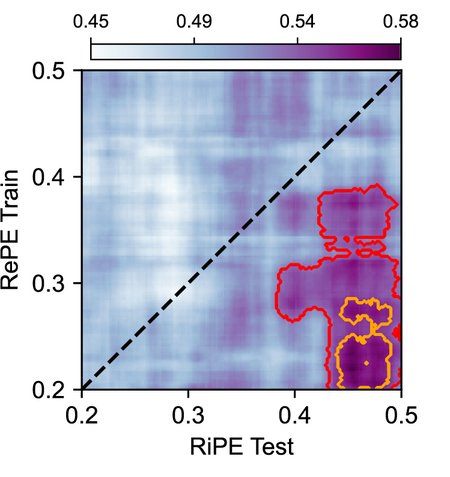